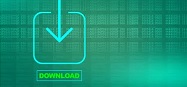

In this review, we describe the applications of radiomics and radiogenomics from the perspective of neuroradiologists, neurosurgeons, and neuro-oncologists. The integrated study of data from radiographical and the genomic scales is termed radiogenomics. Radiomic features have been shown to identify genomic alterations within tumour DNA and RNA.
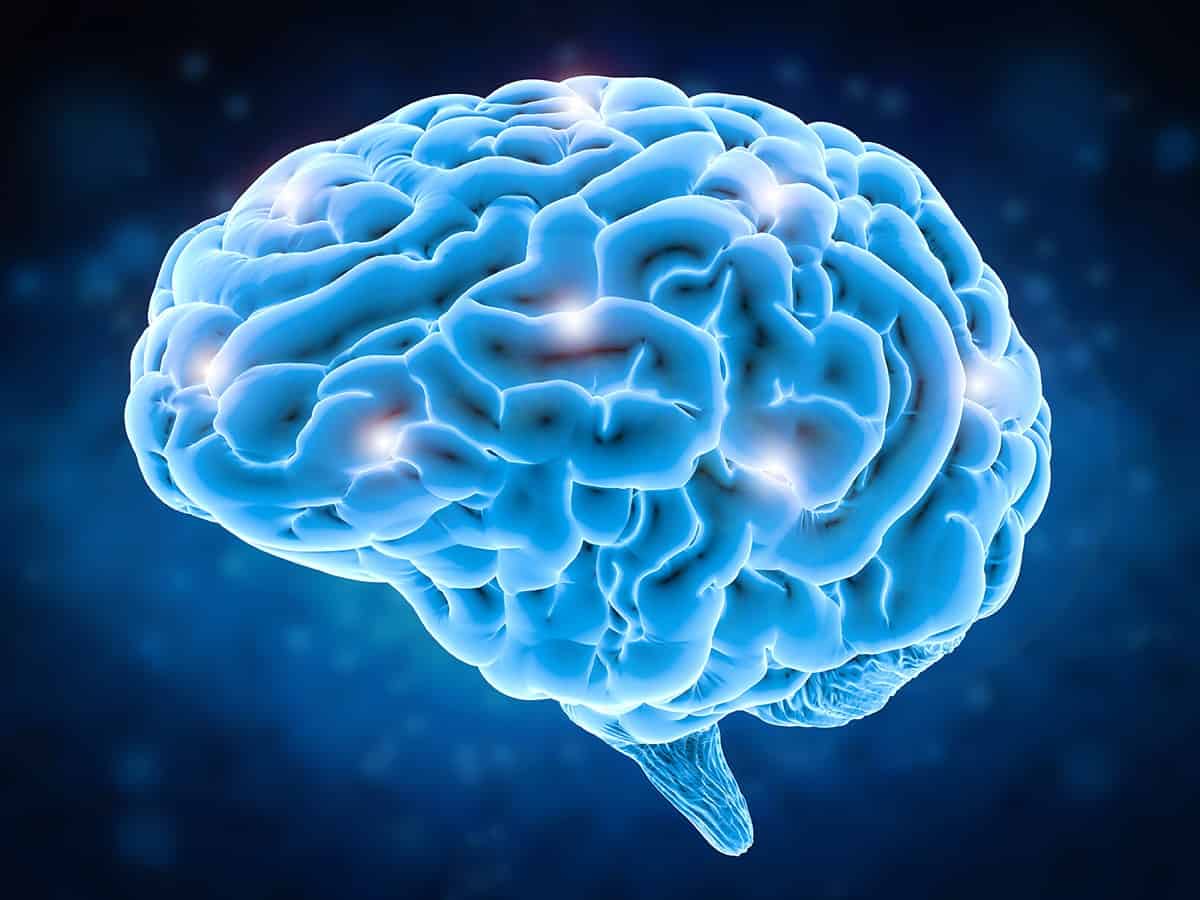
19, 20, 21 Genetic mutations often determine the aggressiveness of the tumour and have been shown to be associated with a lesion’s growth pattern and response to therapy. 17, 18 The extraction of these data creates mineable databases from radiological images which can be used for diagnosis, prognosis characterisation, and to assess or predict response to certain therapies. Radiomics refers to the computerised extraction of quantifiable data from radiological images in the form of radiographical cues that are usually sub-visual. 11, 12, 13, 14, 15, 16 The limitations in current imaging techniques provide an opportunity for more sophisticated sub-visual feature analysis to augment the morphological features and current functional imaging capabilities.
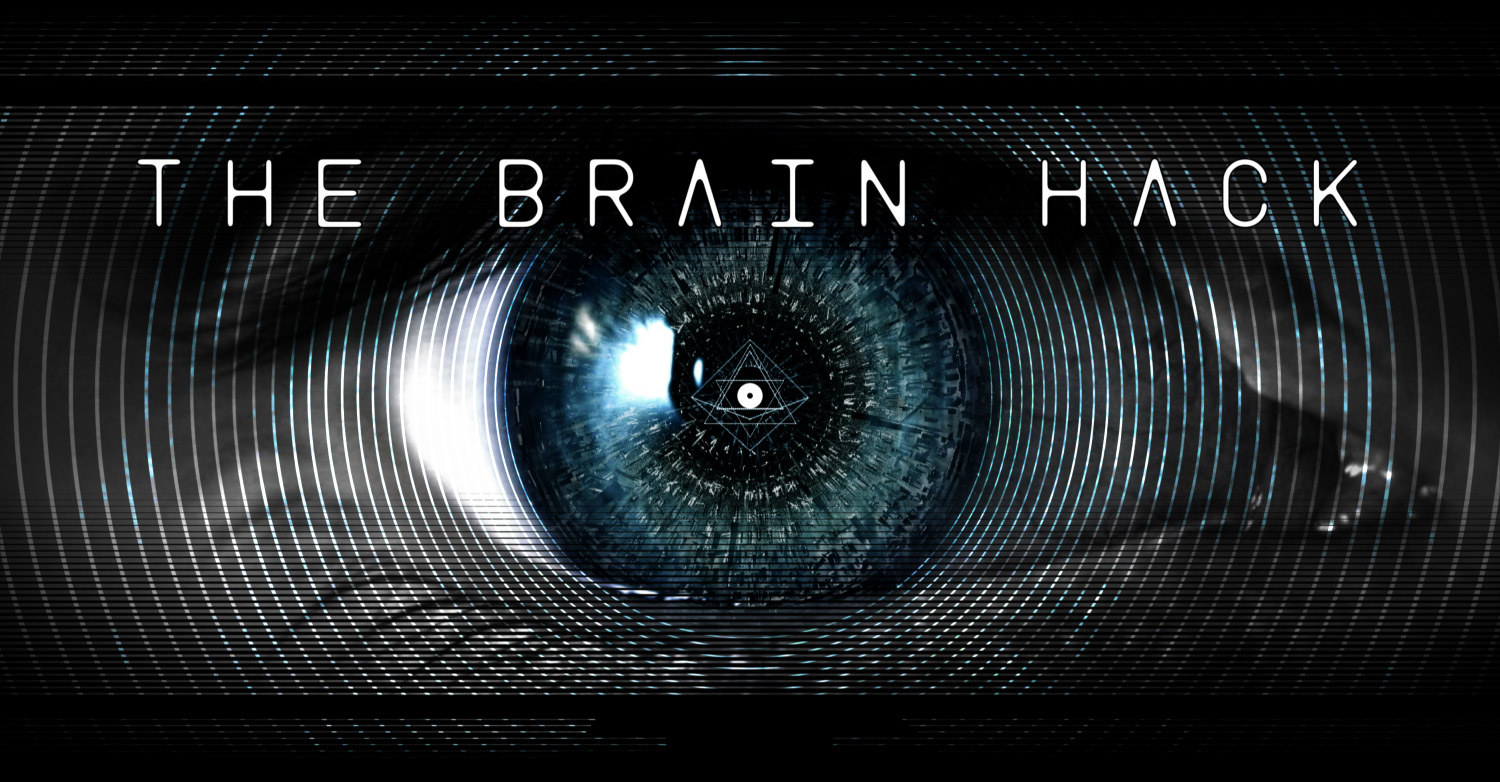
5, 6, 7, 8, 9, 10 Similarly, functional imaging techniques such as perfusion weighted MRI and magnetic resonance spectroscopy (MRS) have been shown to be beneficial when used along with morphological features, but with limited success and reproducibility. Recently, multiple studies have shown strong association between morphological features from multiparametric magnetic resonance imaging (MRI) and survival. 4 This necessitates imaging surrogates to characterise tumour heterogeneity. Stereotactic brain biopsy, despite being invasive and costly, remains the reference standard for histological and genetic classification however, pathological diagnosis may still remain inconclusive in 7–15% of patients. In today’s era of modern imaging, accurate non-invasive prediction of glioma grade/type, survival, and treatment response remains challenging. These changes were incorporated because of the impact genetic factors have on tumorigenesis and subsequent therapy. 3 The new criteria reclassifies entities with the incorporation of genetic information in certain tumours. 2 Previously, the classification criteria was based solely on microscopic features. 1 However, the rate varies significantly by age at diagnosis and the histology of the tumour.Īdvances in our understanding of the molecular pathogenesis of gliomas has prompted significant changes to the World Health Organization (WHO) classification of central nervous system (CNS) tumours in 2016. The age-adjusted mortality rate is 4.4 per 100,000 and the 5-year survival rate is 35%. 1 The incidence is highest for glioblastoma (3.21 per 100,000 population), followed by diffuse astrocytoma (0.46 per 100,000 population). Gliomas account for 80.6% of all malignant brain tumours. Primary brain tumours account for about 2% of all cancers in the US with an incidence of about 23 per 100,000. We elucidate novel radiomic and radiogenomic workflow concepts and state-of-the-art descriptors in sub-visual MR image processing, with relevant literature on applications of such machine learning techniques in glioma management. However, the lack of standardisation of acquisition parameters and inconsistent methodology between working groups have made validations unreliable, hence multi-centre studies involving heterogenous study populations are warranted. This is achieved by a triumvirate of morphological, textural, and functional signatures, derived from a high-throughput extraction of quantitative voxel-level MR image metrics. Radiomics and radiogenomics promise to offer precise diagnosis, predict prognosis, and assess tumour response to modern chemotherapy/immunotherapy and radiation therapy. The natural history and treatment landscape of primary brain tumours are complicated by the varied tumour behaviour of primary or secondary gliomas (high-grade transformation of low-grade lesions), as well as the dilemmas with identification of radiation necrosis, tumour progression, and pseudoprogression on MRI.
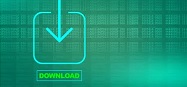